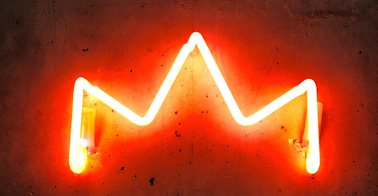
As AI and LLMs move into common use outside of tech communities, many learning and development professionals are asking themselves how this will impact staff development, especially for junior or entry level employees. When is AI the most efficient choice to solve a problem, and when are skilled subject-matter experts irreplaceable? How can L&D leaders reap the benefits of technological progress without burning out, or pushing out, talent?
This piece will discuss the real capabilities of AI models, its impact on existing staff and new hires, and strategies for staff retention amid concerns that it will replace all or part of their job.
For clarity’s sake, let’s talk about what these terms actually mean. AI officially stands for artificial intelligence, and is colloquially used to describe the outputs of Large Language Models (LLMs).
The difference between a true AI and an LLM is the ability to come up with new ideas from an existing data set. An LLM is a compiler of existing information, but it cannot create insight from that information. While many people use AI to describe the outputs of an LLM, the actual results are closer to a blurry JPEG of the internet. In this article, we’ll be using AI and LLM interchangeably.
When a company decides they’re going to build an LLM, they need data sets to train it on. These data sets are bits of information that are given to the LLM to learn from. The largest data set, called the Common Crawl, contains 12 years of internet data, including “raw web page data, metadata extracts and text extracts.” The ethics of this data set have been hotly debated, especially since websites were not always aware their data was being collected.
As more users adopt ChatGPT, OpenAI and other LLMs, concerns about the technology have intensified. In many cases, these issues parallel previous adoptions of emerging technologies and historical trends.
Like any new technology, LLMs have their limitations. Knowing those limitations, and understanding how they can impact your team, is vital to successful adoption. AI is the latest of many “game changing” technologies we’ve seen over the last several decades, and it certainly won’t be the last. Understanding how new technologies will impact your team is key to successful adoption.
The promise of AI is that it will make writing, coding, planning, facilitating, and many other aspects of knowledge work easier and faster. With all of these options, there’s a lot of pressure to start incorporating AI into your day to day operations to stay current on technology. In certain situations, it can absolutely save time and resources for your team. However, it only lives up to that promise under the following conditions:
In reality, AI thrives under a different set of conditions:
For example, LLMs can be great for first drafts where the information is already known or can be considered common knowledge. Marketing professionals tout them as an easy way to write blog entries and webcopy. They can also be useful in coalescing existing information into a plan.
For example, education firms are incorporating generative AI into their offerings with claims of personalized learning experiences. Using AI to build a draft chatbot for your website is a great way to answer common client questions, provided there is a way to get to an actual support person for issues that cannot be easily resolved.
The same thing can apply to building new policies. The AI can pull data from other similar companies, create a template, and then you adjust the information to be accurate to your company. Here at Outgrow Your Garage, our Privacy Policy started as an AI draft, and I made a few tweaks to ensure it meshed with my company values. The downside to this method of creating is that as more and more of the internet becomes AI generated, the ensuing feedback loop is corrupting the base LLMs.
Addressing these limitations with your staff is key to success. AI models aren’t capable of replacing human intuition or experience. At best, they’re able to generate content at the level of a mediocre entry level employee, (one might even say an intern) provided the person editing the text is experienced enough to catch any errors.
AI is still very new, and has changed drastically over the last several months. Its key function is to give the most plausible response to a set of queries, which sometimes means it “makes up” information.
It doesn’t matter whether AI will make your work life easier in the long run if there isn’t an adoption and implementation plan for success.
Whether you’re adding professional development opportunities or introducing new technologies to your team, it’s vital to ensure time in the schedule for learning. Asking employees to take on new responsibilities without accounting for the time those responsibilities will take increases the likelihood of overworked and burned out staff. While the promise of AI is to reduce workload, we’ve seen similar promises fail to pan out in the past.
When email was first introduced, if offered the utopian promise that paperwork would be reduced, communication would be faster, and workplaces would become more efficient. While all of those things have certainly happened, there hasn’t been a comparable drop in workplace hours to offset the rising efficiency. Work hours keep rising, leading to faster rates of burnout among employees. Communication overload actually makes it harder for employees to prioritize and get tasks done, which is the exact opposite of the future promised by early email aficionados.
As an organization, you can avoid this fate for AI or other emerging technologies by considering its real impact on someone’s workload. It doesn’t matter whether AI will make your work life easier in the long run if there isn’t an adoption and implementation plan for success. Questions to ask could include:
A skilled worker will be able to evaluate the question for nuance, ask for clarifying information if needed, and then provide a best fit answer.
One of the biggest promises of automation is the promise of lower payroll costs. With AI specifically, there’s an increased push to rely on the technology for answers instead of skilled workers. In recent months, it’s likely that anyone reading this article has seen some variation of the following interaction:
Person 1: Does anyone have a recommendation for solving this problem?
Person 2: Did you ask ChatGPT?
Person 3: In my experience, I’ve done x and had these results.
Person 1: Thank you!
There are a few things that are happening in the above exchange. Person 1 is asking for help in a specific email chain, slack channel, or other place where someone who knows the answer might be. Person 3 offers their experience to help Person 1 with their query, and everyone goes on with their day.
Person 2 likely wants to help Person 1 find the answers they’re looking for, but they’re disregarding something crucial: this question was posed to skilled workers. LLMs will provide the most likely answer to a given question, but the most likely answer isn’t always the best fit. A skilled worker will be able to evaluate the question for nuance, ask for clarifying information if needed, and then provide a best fit answer.
Consider this graph. Because LLMs are trained to predict the most likely outcome, they will typically provide answers within the middle two sections of a bell curve. Someone who has knowledge of a particular field or subject matter is much more likely to offer a solution on the far right. They are better able to disregard irrelevant or incorrect information through source evaluation skills, and so can provide a better answer.
As your organization adopts AI-based solutions, part of the implementation process will need to account for understanding when AI is an appropriate tool and when you need the help of an expert. Recognizing that it cannot replace your skilled staff creates a greater chance of success within your organization.
What about the other side of the employment spectrum? Entry-level positions are often full of rote tasks that can be potentially adopted by AI, and technophiles have long claimed that new technologies will lead to increased jobs. However, the promised jobs haven’t actually appeared. Entry-level jobs are a vital part of growing a skilled workforce, and using AI to replace these jobs will make it harder to find skilled workers in the future.
We’ve seen this issue play out in the skilled trades over the last 20-30 years. As more US high school students were directed to college, the number of people entering the skilled trades lessened. Now, there are plenty of high-paying trades jobs, but not enough workers to fill them.
AI can certainly replace elements of an entry-level job. Collating data, answering simple questions, and doing basic research are all well within current AI capabilities. However, an AI doesn’t learn the ins and outs of your organization, nor can it be trained to evaluate information. It cannot grow into a skilled employee.
As technology advances, more and more tasks may be able to be offloaded to an AI, but it’s important to balance that with creating ways for entry-level employees to grow into tomorrow’s skilled workers.
Creating space for new technology adoption, training existing staff in its use, and ensuring a steady flow of new hires to replace those leaving can seem like a daunting task. Luckily, the solutions to those challenges overlap. Ensuring regular time for professional development means that when a specific training measure needs to be implemented, the time is already blocked out. A learning organization has curiosity baked in, so staff are more likely to be interested in discovering how new technologies like AI can make their job easier.
Hiring entry-level employees and providing upward mobility opportunities is one of the easiest ways to retain millennial staff and attract Gen Z. Once they’re hired, you can incorporate the same methods of professional development to grow their skills.
Mentorship programs in particular are a great way for new entrants into the workforce to learn from your more experienced staff. These initiatives can be extremely low cost, and increase the feeling of community at your organization. Mentors feel valued, and can pass their knowledge and skills onto the next generation. Mentees can learn from those experiences, as well as receive individualized training.
Paid internships are another way to introduce entry-level staff to your organization. They’re like an extended job interview, where you can answer questions around existing skills and culture fit, but without the hiring risk. Internships can be as short as a few weeks or as long as a year, and the National Association of Colleges and Employers has a great list of best practices to get you started.
While those in the Learning and Development communities are often already proponents of these measures, it can be hard to convince those in upper management or the C-Suite of their value. However, programs targeted at growing internal staff skills don’t solely benefit new and entry-level hires. Professional development opportunities increase staff retention at all levels. And if you’re putting in the effort to train someone, then you want to reap the rewards of their newfound knowledge - whether you’re training them in equitable AI use or looking for your next department head.